A Strategy for Predicting How Long It Takes for ELL Students to Reclassify
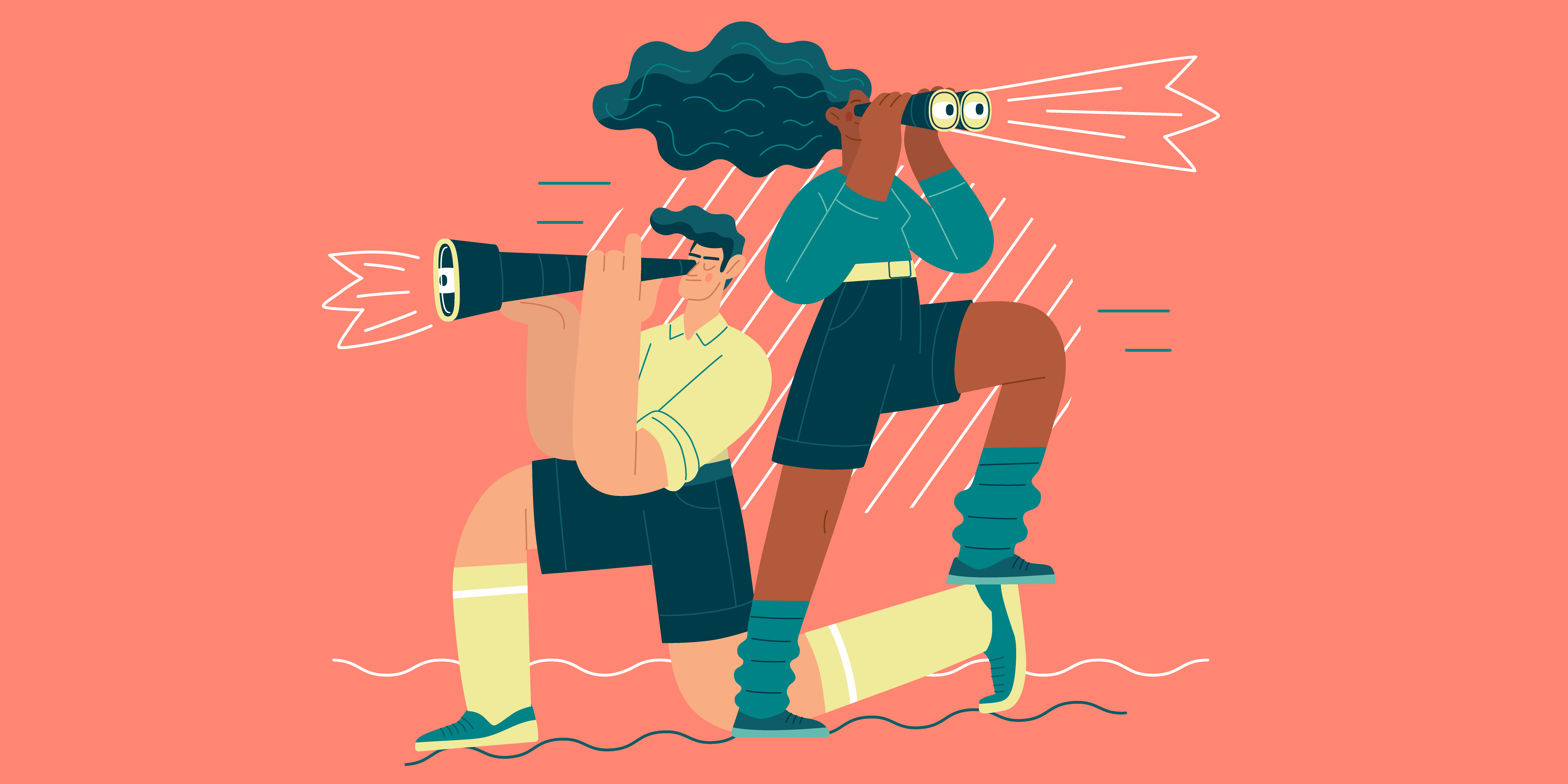
When a new research study is released, it is natural for education practitioners to want to go straight to the findings to see what’s most useful. With the publication this week of our new REL Northwest English language learner (ELL) study, we think districts have much to gain not just from the findings but also the methodology we used to arrive at our findings, which districts can replicate to serve multiple purposes.
Our study was requested by the Road Map Project, a cradle-to-career collective-impact initiative involving seven Seattle-area districts, community-based organizations, institutes of higher education, and other stakeholders. More specifically, we worked with Road Map’s ELL Workgroup who wanted to learn more about the students they serve and the time it takes for their ELLs to reclassify—or in other words, how long it takes for students to pass a language proficiency test and exit ELL services.
One approach to answer this question is by looking at classification dates, gender, home language, and race/ethnicity for several thousand current and former ELL students in the participating districts and then calculating an average for how long it took the students to reclassify. (This is the process one of the authors used in a previous REL Northwest study published by IES last year.) A second method—and the one we used for the new study—employs survival analysis, which is a predictive rather than descriptive model, to determine how long it will take for individual ELLs to reclassify based on student characteristics.
While calculating an average provides a snapshot of a specific sample and can provide useful information, the findings are not generalizable to evolving populations over the course of time. However, survival analysis, because it is a predictive, provides an opportunity to reconfigure expectations when the composition of student populations shifts.
For example, one of our findings is that English learner students entering kindergarten with advanced English proficiency were more likely to be reclassified in their first eight years of school than those who enter with basic proficiency. In the event that demographics shift and a district has an influx of students entering kindergarten with advanced English proficiency, survival analysis teases out student differences and lets the district predict how long students sharing the same characteristics will take to reclassify. This can inform processes such as program planning and resource allocation.
From our experience, districts don’t often use a survival analysis method because they are not aware of it, their data analysts are mostly focused on federal reporting, and the methodology is technical and could be beyond the capacity of many districts to properly and efficiently utilize it. These obstacles are legitimate, while at the same time, the benefits of using student data to predict future needs are also very powerful. For districts interested in learning more about survival analysis, we offer these considerations:
Form a Researcher-Practitioner Partnership
In keeping with the national emphasis on researcher-practitioner partnerships, a researcher can help districts become more critical consumers of data. Together, districts and researchers can create a predictive model that will help not only track progress of specific student groups but also inform planning as student demographics shift. A researcher can also help build a district capacity to run data through this methodology on its own.
Consider Other Applications
Survival analysis is flexible and can be applied in different scenarios that result in specific events. In our study, we used ELL student reclassification as the event around which we built our model. This methodology can be used in other areas such as for early warning systems based on events such as dropping out of school, high school graduation, and others. While survival analysis was developed by medical researchers to determine the effectiveness of various treatments, it’s a methodology that has multiple applications for districts wanting to use data to create a predictive tool.